CPOS Seminar: "Beyond Molecular Structure – Machine Learning for Designing Organic Photovoltaic Materials and Devices"
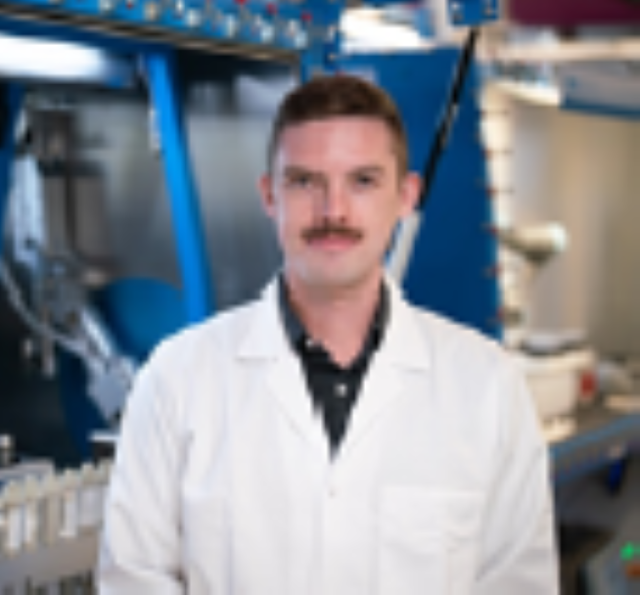
Speaker:MARTIN SEIFRID, PhD, University of Toronto / Aspuru-Guzik Group
Abstract: Organic solar cell (OSC) materials are a class of organic semiconducting molecules designed to convert sunlight to electrical energy. OSCs are typically constructed by blending two or more of these materials together in solution, and then forming a bulk heterojunction active layer within a layered device architecture formed by additional electrode and interlayer materials. Different materials and combinations thereof, solvents, concentrations, and processing conditions create an experimental space that is essentially infinite. Developing new OSC materials and optimizing their performance is a time- and resource-intensive process. Therefore, the OSC community is beginning to look towards data-driven techniques, such as machine learning (ML), to greatly reduce the time and effort required to increase device performance.
We will discuss how the OSC community can benefit from rigorous implementation of ML models. To do this, we have investigated the impact of including key processing and device fabrication parameters on ML models as an opportunity to examine the ML process as it’s applied to OSC research. We have trained predictive models using well-established and proven ML algorithms to allow chemists to design new materials and device architectures with higher power conversion efficiencies (PCE). Furthermore, we will be publicly releasing a large dataset of OSC materials and devices, focusing on key metrics of performance that have been excluded from previous efforts. We will focus on important aspects of ML model building and implementation including: (i) dataset curation, especially as it relates to literature data in inconsistencies therein, (ii) appropriate selection of ML models and representation of molecular structures, (iii) feature engineering, and (iv) model training. Finally, we will highlight what can be learned from the models that we have implemented to provide guidance to chemists, materials scientists, and device engineers seeking to increase PCEs and better understand the scientific questions still facing OSC research.
Bio: Dr. Martin Seifrid is a postdoctoral fellow in Prof. Alán Aspuru-Guzik’s group at the University of Toronto. His research focuses on the development of self-driving labs for organic materials design and discovery, and the application of machine learning to materials for energy applications. Prior to arriving in Toronto, Martin completed his PhD in CPOS, where he studied the temperature-dependent behavior of organic semiconductors